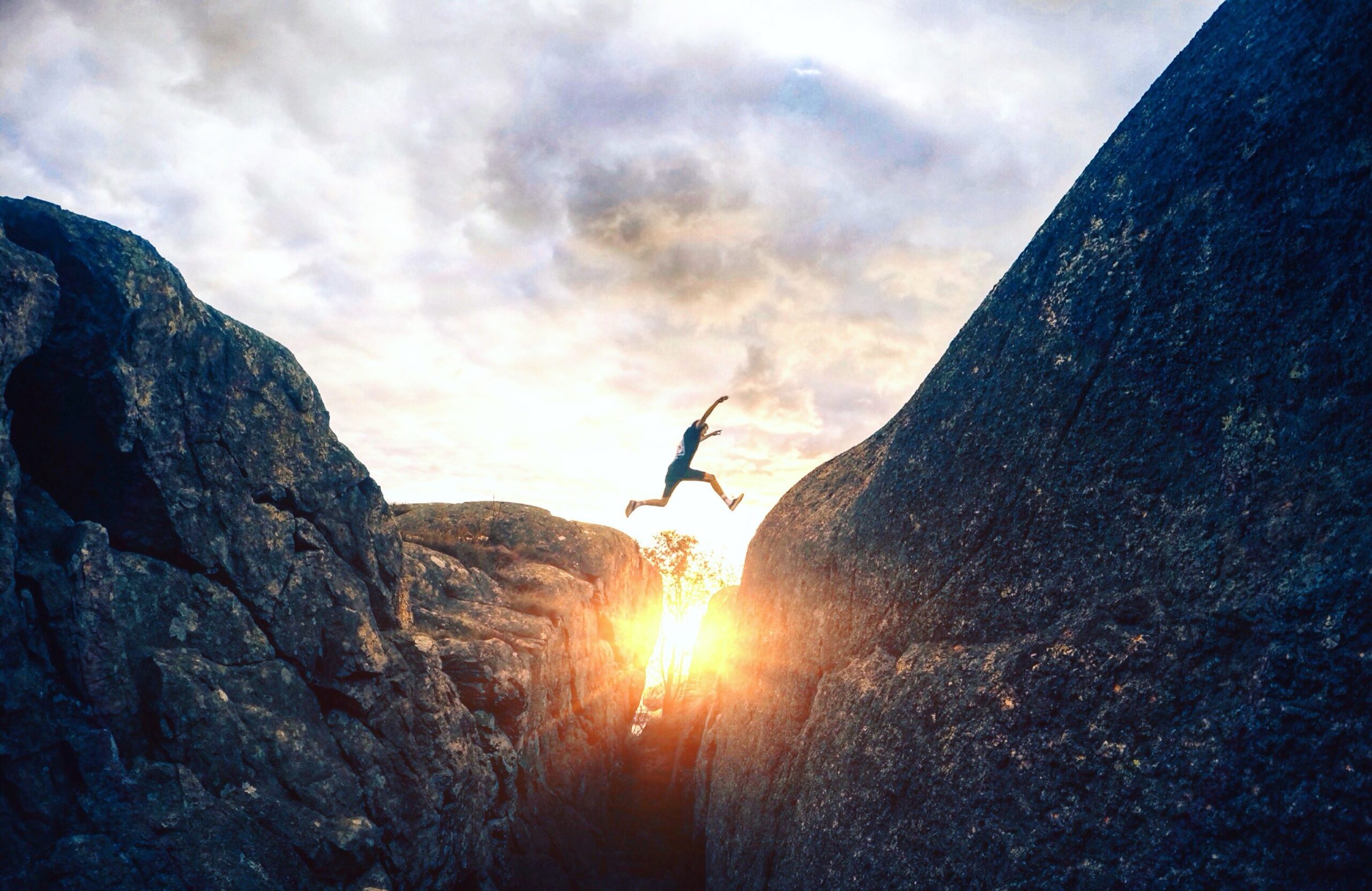
Seems like the technology industry—including enterprise analytics—is always in search of the latest buzz phrase about which to get excited. Internet of Things. Digital transformation. Big Data. Artificial intelligence. You get the idea.
And it’s not necessarily bad. Technology changes at a dizzying pace, and the velocity with which we generate and consume data is almost ineffable. The problem with using buzz phrases is not the new concept, platform, or product to which it applies; rather, it’s that we often forget what’s fundamental about the concept being discussed while focusing on what’s new. And that can cause problems.
Look at business intelligence (BI). The popular buzz phrase of the BI world is “analytics.” And most people assume that buzz phrase refers to data collection and reporting. Yet fundamental analytics goes beyond simply collecting and reporting on data, and speaks to the greater insights found by observing patterns in the information—i.e. “analyzing.”
Unfortunately, while every company wants to be better at analyzing data, many simply don’t have the foundations in place to do so. Using legacy, oppressively expensive approaches like a data warehouse and data modeling, users receive diluted information that’s radically different from the detailed data initially captured. Users futilely try to analyze—surmise patterns in—this diluted information, arriving at incorrect conclusions or misguided insights.
The result? They don’t trust their conclusions or insights because they don’t trust the data upon which they’re based.
In this case, the fundamental truth the buzz phrase “analytics” fails to adequately communicate is this: before you truly can analyze it, you have to be able to trust the data.
I call it “data trust.” That’s my new buzz phrase. And, unlike data governance or data cleansing—which refer to trust in the process that provides information (and albeit is equally important)—data trust is about easily accessible transparency.
You’ll have to Live Without Data Trust if You Use a Data Warehouse
Data trust occurs when the users, analysts, and executives in an organization easily and quickly can access details that support the transactions they see—they can drill down into the details to understand the underlying context or to validate their assumptions.
Access to those details—those transactions—is the foundation of data trust. Without access to the details, your user community can only guess (or hope) that what they see is correct. They can’t get at the truth behind the patterns. And that approach—what happens when you use a data warehouse—doesn’t inspire trust.
For example, the scatterplot below displays sales trends across regions for cell phone models. The data shows one region and model in particular is performing poorly, based on marketing spend.
That pattern definitely deserves further analysis. What exactly is happening? Why is it happening? Is there anything that can be done to quickly correct it?
The real answers lie in the detailed transactions. But how do you dive into the details if you’re using a traditional, data warehouse-based approach to analytics? You can’t. You can try to create a series of additional reports that review marketing spend by region—high-level sales reports that drill into the data, down to the retail location or sales person. But there’s no guarantee rigid reports like these will give you the answers you seek. And they definitely won’t enable you to drill down into more details as you see fit.
The same problem occurs with financial statements. How can you determine the specific transactions feeding into the total cost of sales metric shown below? Ideally, accessing that type of detail should be a single-click, but it often isn’t possible for a user to ever access that kind of information.
At a certain point, traditional analytic systems simply run out of detail. That’s because they require you to abstract and reshape the data: what starts out as a series of transactions and information spread across your enterprise resource planning (ERP) or General Ledger (GL) systems quickly becomes—for example—10 tables in a data warehouse or a few cells in a cube.
When you want a new piece of information for analysis, your IT team needs to extract that information from the original data source, convert the information to match the structure of your data warehouse or data cube, and then map it into an unnatural structure. This common approach further abstracts the data, becoming yet another building block in the wall separating you from the detailed data—the source details—you need for transparency and trust.
Source details are the only real truth in the data. They explain every pattern. When these details are missing, users relying on traditional systems hit the wall when they try to understand patterns. They end up requesting detailed transactional information from the IT organization (or using secondary operational reporting tools) to ensure what they see is supported and to try to determine the root cause.
Without source details, they’re unable to focus on the patterns. And the patterns are the true definition of “analytics.”
Inspiring Data Trust: A New Approach to Enterprise Analytics
Traditional enterprise analytics practitioners will tell you this problematic approach is unavoidable and the best they can do because:
- ad-hoc analysis on transactional data is inconceivable because the data you need is spread out across sources and structures;
- moving data directly from table to table within a database does not work; and
- you need to do it this way for performance reasons.
But let’s take a fresh, unbiased look at how we could achieve this nirvana of data trust: if you wanted to close the gap between observation (pattern recognition) and proof (details), how would you do it?
The answer is as obvious as it seems: work directly with the source data and generate your analytics directly from it.
If you don’t have to model and reshape data—if you knock down the wall and instead build a bridge between analytics and supporting details—you will save enormous sums of money on the implementation, and your organization will be able to analyze and validate data patterns, then quickly take appropriate actions in response.
In short, you’d have data trust.
Contrary to traditional belief, modern data platforms that allow you to handle data in this way do now exist. They allow this type of data access and also deliver sub-second response times on hundreds of millions or billions of rows of detailed, transactional data.
No longer do you have to abide by the legacy paradigm of data warehousing and data modeling, with complex reshaping of the data into star schema, cubes, and so forth. No longer are you forced to place a risky, million-dollar bet you’re likely to lose.
It’s worth exploring a better way—a different way—that builds trust in the results you see.
Want to learn how Incorta inspires data trust? Contact me directly at mike.nader@incorta.com.