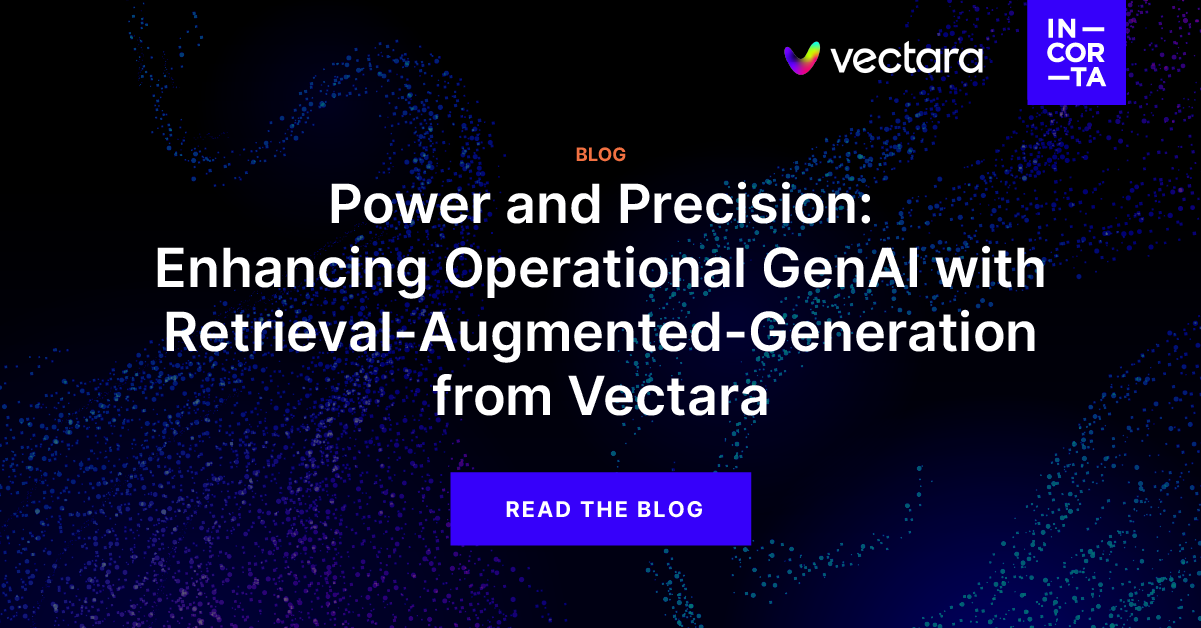
According to a recent MIT Tech Review report, all 600 CIOs surveyed stated they are increasing their investment in AI—71% are planning to build their own custom Language Learning Models (LLMs) or other GenAI models. Despite this, many organizations struggle to successfully implement their GenAI initiatives because they lack access to live, up-to-date data for their models.
Successfully deploying GenAI models to any area of your organization requires a strong data foundation – with access to fresh, detailed data while preserving security and compliance. For Incorta’s first Operational GenAI offering, Nexus, we’ve joined forces with our strategic partner Vectara to integrate their state-of-the-art Retrieval-Augmented-Generation (RAG) capabilities – helping organizations mobilize their GenAI initiatives more accurately than ever.
Effortlessly gleaning insights from structured – and unstructured – data with RAG
Access to live, detailed, operational data is critical when deploying GenAI initiatives. However,
unstructured data poses a challenge for companies aiming to leverage GenAI – the sheer volume and diversity of this data can make it difficult to glean any actionable insights.
When applying GenAI, language models must prioritize specific organizational data for reasoning tasks. This is where Retrieval-Augmented-Generation (RAG), powering Incorta Nexus, significantly boosts AI-generated accuracy, relevance, and reliability.
Vectara’s trusted Gen AI platform allows organizations to rapidly create AI assistants and agents grounded in their data, documents, and knowledge. Vectara’s serverless RAG also provides the critical trust and control capabilities required for enterprise adoption.
By leveraging high-quality, consistent, and real-time structured data from Incorta for model training and performance and Vectara’s RAG capabilities for access to relevant unstructured data, organizations can enrich their AI’s contextual understanding and response generation for better accuracy.
- Vectara for Retrieval:
- The retrieval component uses Vectara’s advanced hybrid search capabilities and Boomerang Model to find the most relevant information. This is based on understanding the context and intent behind the user’s query.
- This step ensures the generative model can access accurate, diverse, and contextually appropriate information.
- RAG:
- Once the relevant information is retrieved, it augments the input to the generative model. This means the model can access a focused set of relevant data rather than relying solely on its training data.
- This augmentation helps the model generate responses that are more informed and accurate.
- Vectara for Generative:
- The generative component\ uses the retrieved information to construct a coherent and contextually appropriate response.
- This approach allows the generative model to produce more precise and contextually relevant responses than it would if operating in isolation.
Using a RAG model, Incorta Nexus is elevated with:
- Enhanced Relevance: By leveraging retrieval to inform generation, responses are more relevant and accurate to the user’s query.
- Contextual Awareness: This approach allows the model to incorporate up-to-date information and a nuanced understanding of the query context.
- Improved Efficiency and Scalability: It combines the strengths of retrieval and generative models and is suitable for handling large volumes of queries and data, making it an ideal solution for enterprises with complex data sources.
Merging the power of Operational GenAI with the precision of RAG
Vectara’s RAG approach combines the precision of retrieval with the flexibility of generation, resulting in a powerful tool for various applications that require accurate and contextually relevant information retrieval and response generation. This is particularly useful for cases like:
- AI Assistants: Vectara’s superior language understanding ensures that AI Assistants can go many layers deep.
- AI Agents: Vectara uses hybrid search to find the most relevant results, products, support cases, and documents that answer your users’ questions first.
- Question and Answering Systems: Vectara helps find summarized answers with citations to guide your decisions
Nexus Through the Lens of a CFO: Enhancing Profitability Margin Analysis
As a CFO, you are responsible for your company’s profitability margin. If there’s any noticeable decline in the operating income margin, it’s up to you to determine if this decline is attributed to internal inefficiencies or external market factors.
Traditionally, this analysis involves extensive, time-consuming, and error-prone manual work, including data extraction from various operational systems, manual calculations, and scouring available competitors’ financial reports.
Using Incorta Nexus, CFOs can leverage the platform’s ability to integrate and analyze structured and unstructured data in real-time. Here’s how a CFO would utilize Incorta Nexus to tackle this challenge:
- Initial Query on Operating Income Margin:
- The CFO asks a simple question about the operating income margin for the last two years.
- Incorta Nexus processes this query by scanning all relevant operational data and returning the required information. The trend is displayed in a clear, easy-to-understand chart.
- Adding to Dashboard:
- The CFO adds this chart to a dashboard with a single click, enabling easy access and ongoing monitoring of this key metric.
- Competitor Analysis:
- The CFO asks about competitors’ operating income margins to determine if the margin decrease is due to market conditions.
- Incorta Nexus scours thousands of pages of competitors’ reports, including unstructured data such as PDFs and financial filings, to provide a comparative analysis.
- The results show that a competitor’s operating income margin decreased from 10% to 5%, suggesting a potential market-wide issue.
- Deep Dive into Competitor Data:
- Further investigation reveals the competitor’s margin decrease was due to a one-time $2 billion charge related to regulatory compliance issues.
- This insight is crucial – as it indicates that the competitor’s margin decrease is not due to internal operational inefficiencies.
- Internal Analysis:
- The CFO then focuses on the company’s data – specifically the cost of goods sold (COGS), gross margin, and other operating expenses.
- Incorta Nexus provides a detailed breakdown, revealing that the main issue is a significant increase in compensation costs – which rose from 24% to 31% of sales – overshadowing efficiency gains in materials and freight.
- Detailed Transaction-Level Insights:
- By querying further, the CFO can uncover that the increase in compensation costs was due to higher merit increases and unplanned hires.
- This level of detail is achieved without requiring manual data compilation, enabling the CFO to quickly pinpoint the root cause of margin compression.
With comprehensive insights provided instantly by Incorta Nexus, CFOs can confidently present the findings to the board, highlighting the cause of margin decrease and providing strategic insights – such as reviewing compensation policies and controlling hiring practices – to improve profitability margins.
- Time Savings: The entire analysis, which traditionally takes a week or more, is completed in hours.
- Accuracy and Detail: Access to transaction-level data ensures precise and actionable insights.
- Real-Time Analysis: The CFO can continuously monitor and analyze data, providing ongoing strategic input.
With Incorta Nexus, the possibilities are truly limitless. Through our strategic partnership with Vectara, our customers not only unlock new levels of efficiency and agility but also fortify the foundation for future growth and innovation for future operational GenAI initiatives.
See Vectara’s entire session at Incorta’s NoLimits event on-demand now.