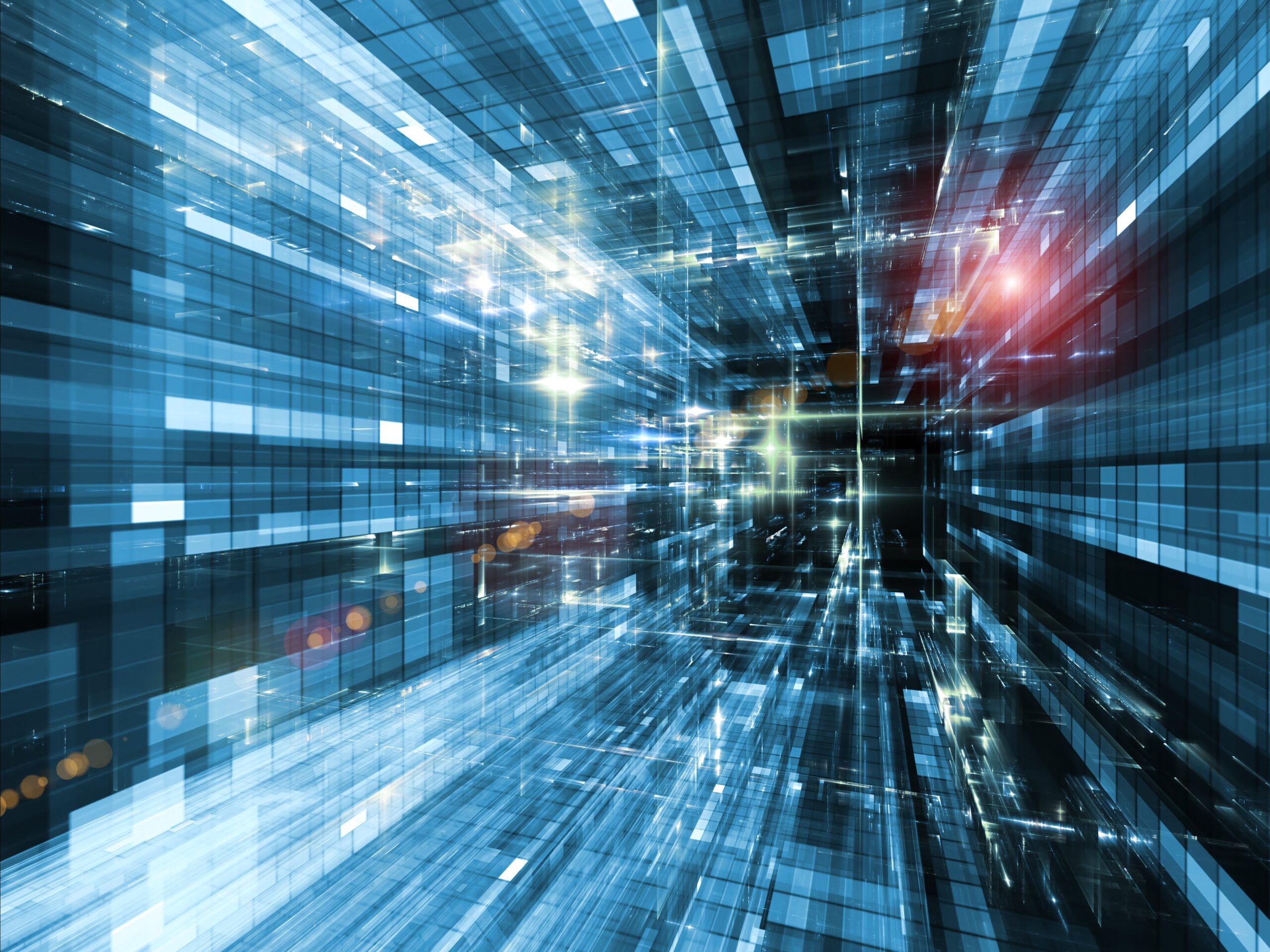
Too often in technology, we treat or talk about the data warehouse as the ultimate step in the analytics supply chain. That position implies putting curated data into a star schema creates all the information required for final reporting and analysis.
But it’s not true. That’s like saying the hardest part of raising a child is bringing the baby home from the hospital. What we forget is that the data warehouse is only the foundation your users need. It’s not the end of analytics: it’s just the beginning.
Somewhere between your data warehouse and the reports your business users need exists that black box of analysis housing additional information and data structures. This cumbersome approach doesn’t give users what they need. And it doesn’t fulfill the promise of enterprise analytics.
Data Warehouses: Rigid but Not Completely Inflexible
Data warehouses serve multiple purposes within an organization: they provide data for user analysis, and they also serve as a clean source for moving data from one operational—or transactional—system to another.
For instance, your data warehouse might feed a cleansed set of sales transactions into a downstream inventory ordering system. In this example, the transfer of data is not for the purpose of user analysis—it’s to drive efficient operations via trusted data.
One of the primary concerns with traditional warehouses is their rigid nature. The business climate around you changes, your needs change, users mature, the rules and structures of the base data warehouse’s original world are no longer realistic—but the warehouse remains rigid. And, often due to fear of changing, we dance with the devil we know: we leave the rigid data warehouse in place and try to work around its limitations.
Now, it’s important to understand a data warehouse no longer is required for successful data analysis. Many modern analytics serve the same purpose without forcing organizations to undertake a traditional warehousing project. Yet legacy data warehouses can become so embedded into the operations of a company, it would take multiple months of impact assessment to even understand the ramifications of removing it. In the end, removing a data warehouse altogether may not be feasible given the cost and effort.
That reality, however, does not mean your data warehouse cannot evolve.
Evolving Your Data Warehouse
To meet user demands, companies modify and expand the data landscape. For instance, a data warehouse designed to accommodate store sales analysis might need to add in a completely new line of business after an acquisition, or it might need to incorporate industry benchmark data due to new business user needs. In many cases, it’s too costly to restructure the data warehouse to accommodate these needs, so many companies instead extend their data warehouse via subject area-specific data marts that fill in that black box of analysis.
These secondary models may perform alternate aggregations, analyze additional data sets, and accommodate new metrics and hierarchies. These types of requirements are critical to the long-term success of the business; but they’re incremental requests not required by the user community when the data warehouse was built.
This traditional approach has two primary problems. First, it’s expensive. You spent millions of dollars building a data warehouse, and then you’ll spend hundreds of thousands—or even millions over time—building additional structures. And these new structures also will require constant maintenance and updating as user needs mature and evolve.
The second issue with the data mart/cube approach is that these structures further reshape data. And each change in data shape further distances users from the original truth of the underlying transactions. Business users need to get closer to their source data, not farther away from it. With reshaping, even if users spot patterns, they have a hard time understanding what caused them.
Ultimately, every business users wants access to the source data, so that data can fully explain what’s observed in aggregations and visualizations. But data warehouses can’t deliver that kind of access—despite their lofty promises.
Modern Analytics: Making Good on a 30-Year-Old Promise
For decades, the world of enterprise analytics has been built upon a foundation of false promises.
These false promises center around the concept of “information freedom”—also known as “end user ad hoc or self-service analysis.” Various technologies and implementation schemes claim to have delivered on it, but all fall short: giving freedom within a 14-dimension cube that’s 10 steps deep is an elegant data prison within a very limited box, not information freedom. And savvy users quickly see the limitations.
This type of restricted approach is based on fear. The tools are complex, the data is extensive, and the users may become frustrated with the experience. So we provide clean data, but we limit their access. And, since it’s so easy to make a “bad” request, we have to dedicate staff to optimizing the underlying analytics systems and the queries that are issued. This convoluted process quickly becomes too expensive and too inefficient. And it doesn’t enable true information freedom.
Fortunately, modern analytic platforms DO give users true information freedom.
- They replace the legacy extensions and allow for far greater freedom—without maintenance and optimization costs.
- They enable enterprises to snap off these incremental subject areas in hours, not days or weeks.
- They push mature users closer to the transactions (the truth in the data), instead of putting additional barriers between them.
These new platforms are viable alternatives to the traditional data warehouse, but they also can augment your existing data warehouse investment. By giving users full, ad hoc, self-service analysis across hundreds of millions or billions of rows—without having to deal with preconceived dimensionality or hierarchies—and receive answers within seconds or micro-seconds, modern platforms deliver true information freedom and remove the fear of data access.
These platforms allow you to simply extend your existing warehouse with additional data as required, then provide what you see fit to your user community for analysis. No cubes are built, and no data is re-shaped into another, more abstracted star schema. In this hybrid approach, the modern analytics platform is an extension of the data warehouse, so it mirrors the nature of that source. And, as your data strategy and user communities mature, you can drive those analyses more toward the source data in those transactional systems to bypass data reshaping altogether.
Modern analytics platforms move us away from the cumbersome modeling that’s plagued the analytics space for decades. Now we can let go of the fear that caused us to put so many restrictions on our data in the first place. We can evolve away from those limitations, toward an agile enterprise, to focus on helping our user community gain true data strategy, analysis and information freedom.
We can finally make good on that 30-year-old promise.
Find out how Incorta makes good on that promise—spin up a free trial today.