Rethink what’s possible with your data.
Whether you need a seamless data delivery solution for Oracle/SAP or a powerful unified analytics platform for your entire organization, Incorta cuts through the complexity of traditional data preparation—delivering fast, flexible, and future-ready solutions.
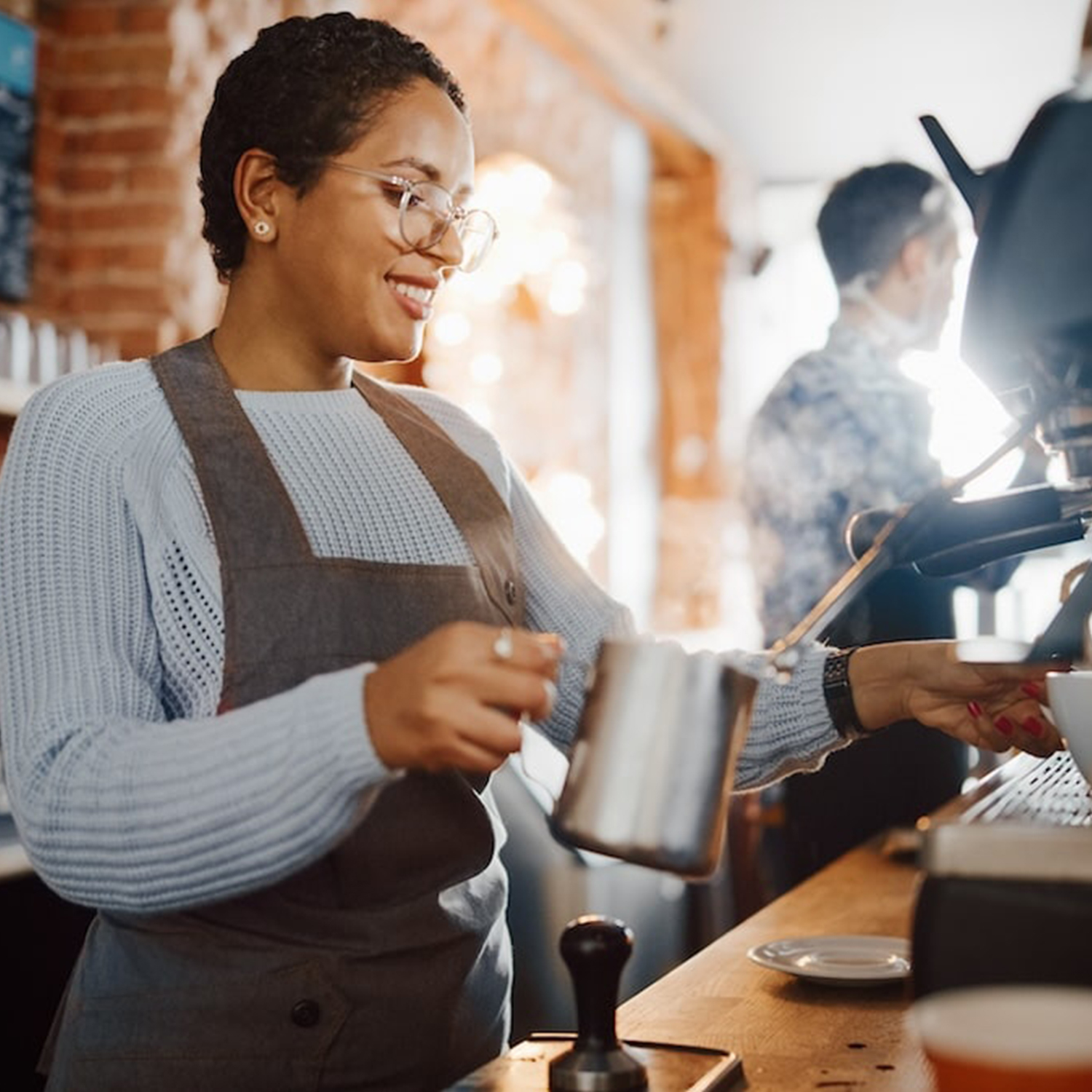
Global Coffee Retailer manages inventory and profitability across 32,000 stores with data that refreshes every 5 minutes.
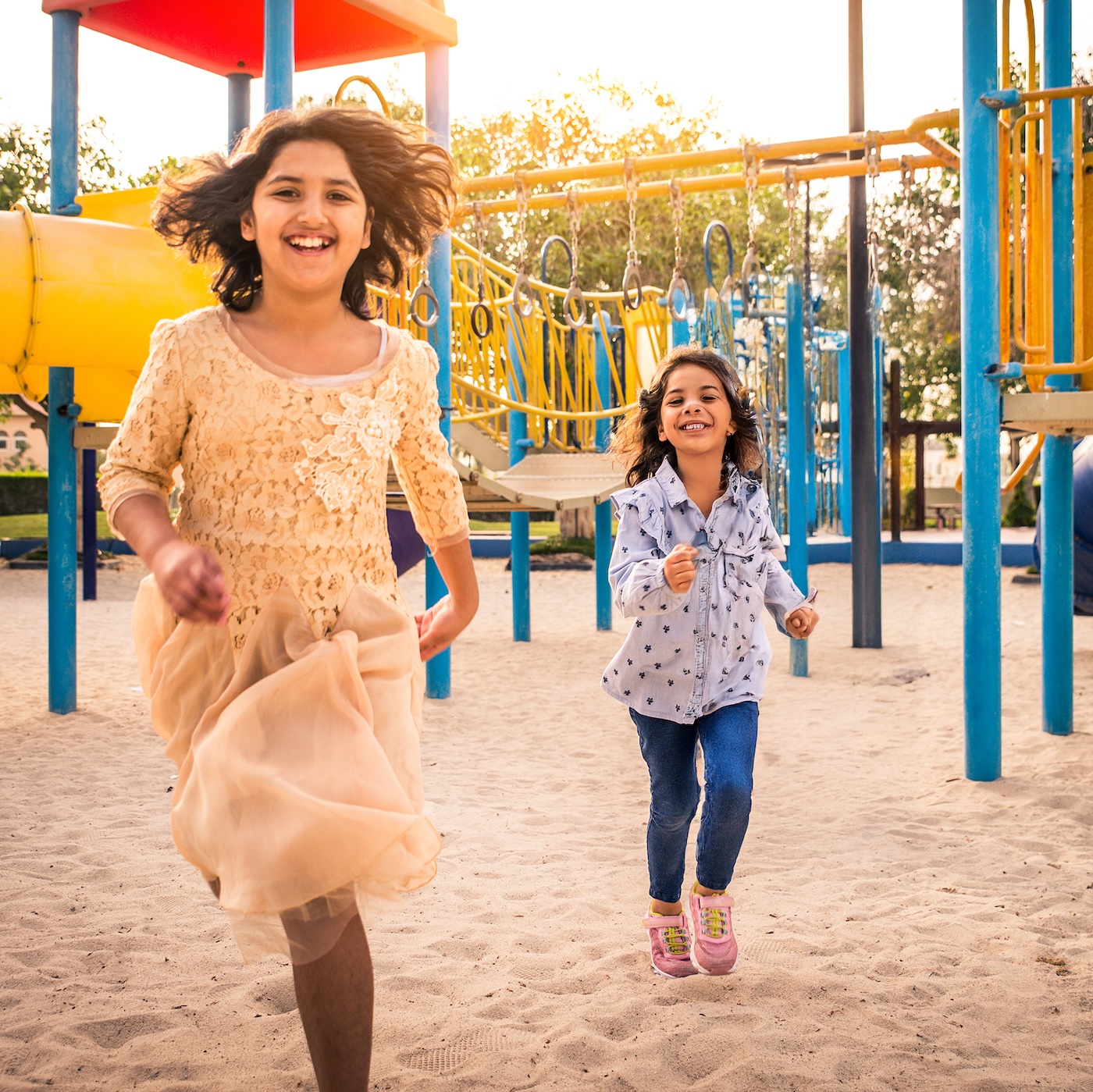

PlayPower users can click on high-level KPIs and drill down to trusted, transaction-level details in seconds.
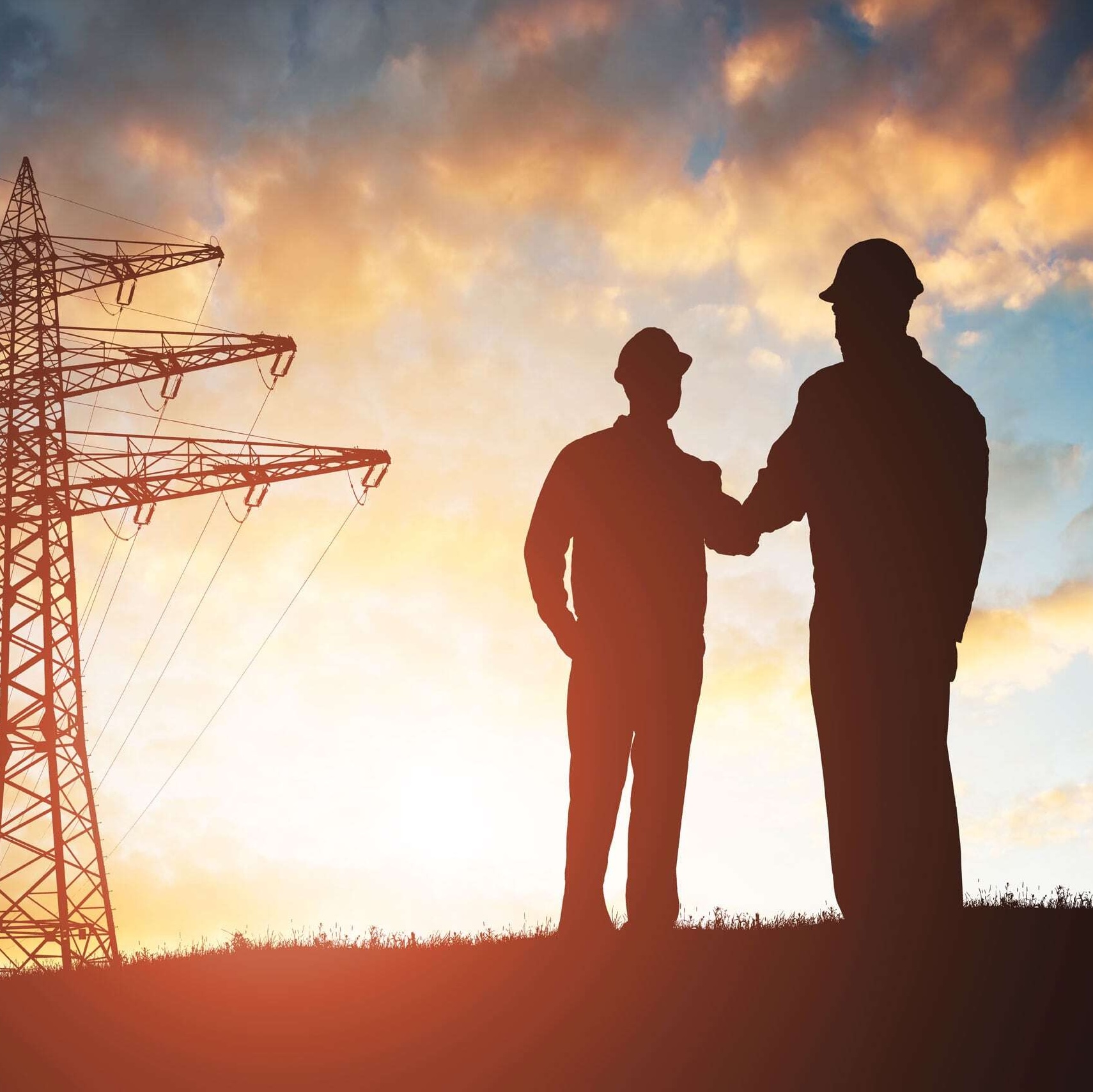
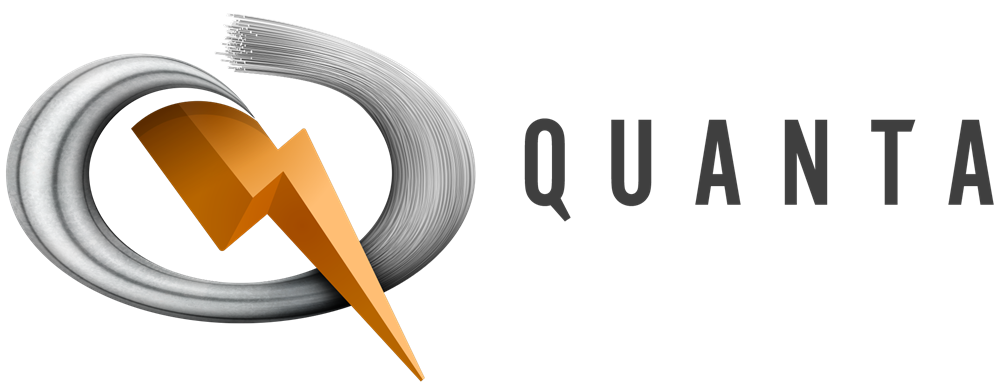
Quanta consolidates data from 70+ ERP systems, delivering unified reporting across multiple legal entities.

Enphase uses fleet-level detail to create analytics-ready data, empowering teams with fast, actionable insights across systems.
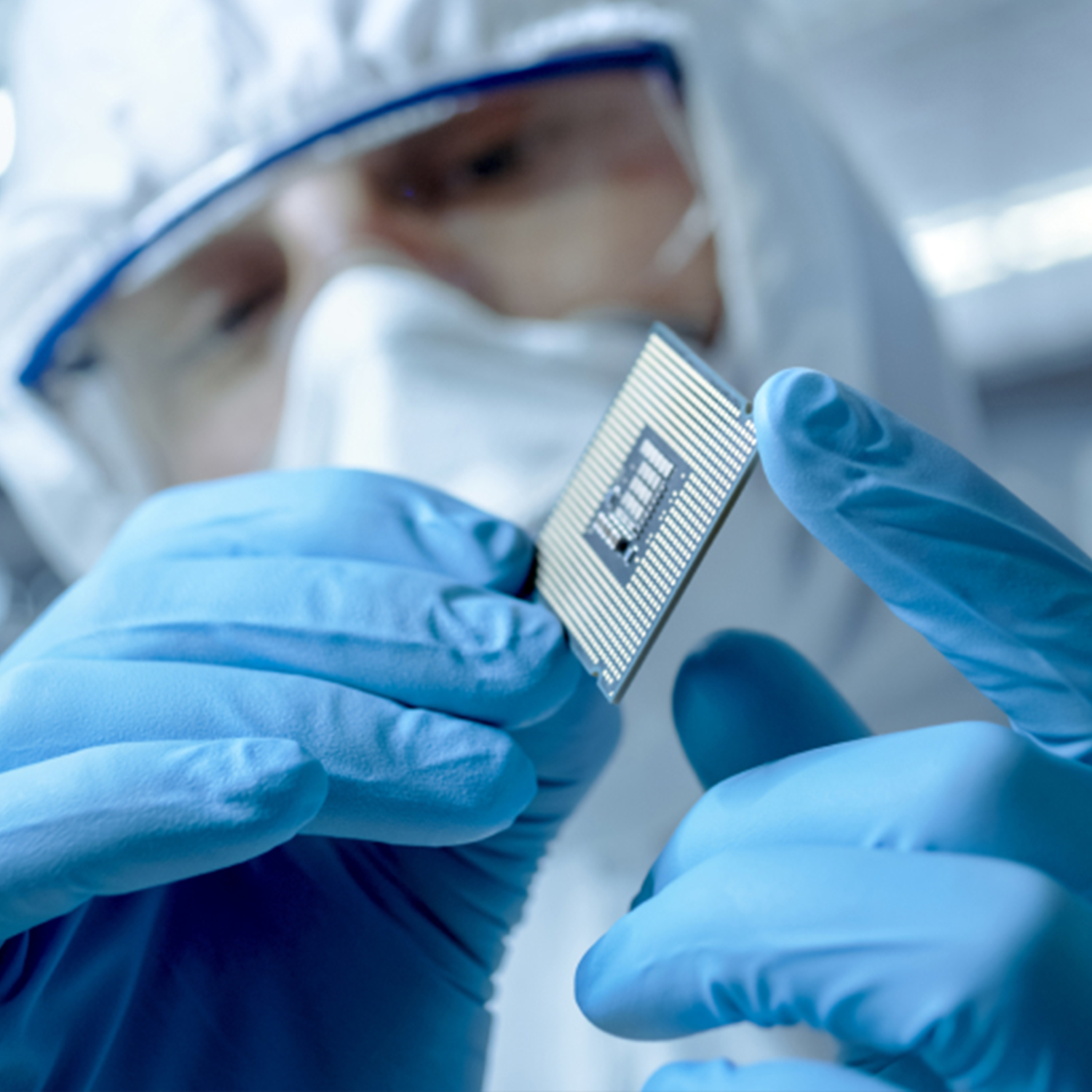